Anatomy of a Data Strategy: From Operational Intelligence to Artificial Intelligence
To get the most from your data, you'll need a comprehensive data strategy. We look at a four-stage maturity model.
- By Abhishek Tandon
- August 12, 2019
The world of business intelligence has transformed over the last decade. As a digital leader, you must understand what to consider when you create a data strategy that delivers business impact.
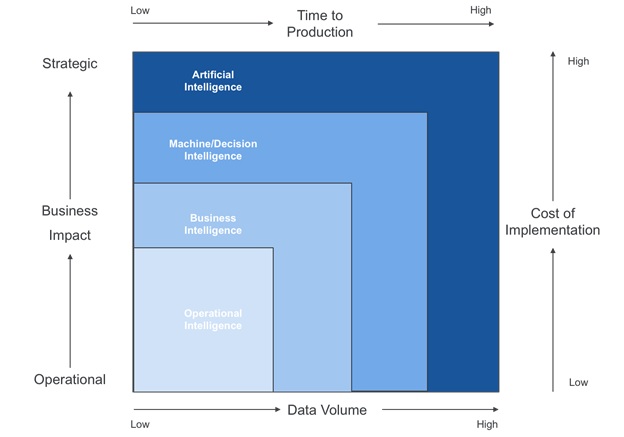
The data cube above highlights the intelligence maturity stages that an organization goes through. The transitions vary on the following factors:
- Business impact: operational versus strategic
- Data volume (available to support decision making)
- Cost of implementation (tying back to business impact and therefore budget)
- Time to production (confidence measure of the decision system)
Every organization looking to build its data strategy needs to start at the lowermost corner and move up the intelligence ladder. The four factors mentioned allow your business to compare impact with investment and decide on the right time to move from OI to AI.
Let's break down each of the categories in greater detail.
The Operational Intelligence Maturity Stage
This is the most tactical-level intelligence needed by lower or middle management to run their day-to-day operations. The time period for reporting is short and does not dwell in the past to derive intelligence. The main purpose here is to know the As-Is scenario. Most of the data that is used by an operational reporting system is sourced from ERP or CRM systems, depending on the type of reporting being done. Data volumes are low; the level of sophistication is also low because the focus is to ensure that business KPIs are being met on a short-term basis.
Impact to business: Low to medium
Cost to business: Low to medium
Consumers: Lower and middle management
OI asks metrics-based questions such as:
- What are the day-, week-, and month-to-date sales numbers in a given territory?
- What is the current production versus plan by region by plant?
- What are the current inventory levels at various steps of the supply chain?
Technologies used typically include some sort of a reporting system either through Excel or a native reporting platform from ERP systems. Customers also tend to purchase low-cost and easy-to-use reporting solutions such as Tableau to build reports for consumption.
The Business Intelligence Maturity Stage
Over time, the need for operational reporting can no longer be satisfied by OI -- it requires decision support systems. More data is generated, which opens up tremendous opportunities to learn from the past. The kinds of questions asked of the data start to change. The time horizon becomes longer and reports generated are increasingly strategic in nature.
Impact to business: Medium to high
Cost to business: Medium to high
Consumers: Middle and upper management
At the BI maturity stage, an enterprise tends to want to compare results or ask about historical trends. Questions might include:
- What were the supply-side to demand-side gaps over the last 12 months?
- Was the issue on the inventory side or production side? If production, which plants led to the increase in the gap? What were some of the issues in those plants that led to an increased gap?
The BI maturity stage requires creating a database that is integrated across multiple functions and is optimized to generate aggregated reports that analyze longer periods of time. Typically, this requires creating a data warehouse. The data warehouse data model takes into consideration important business elements needed for decision making and aggregates data in a way that answers business questions without the need for multiple IT development cycles. From a reporting standpoint, the systems are a mix of inhouse platforms, reporting tools from the past (such as SSRS, Microstrategy, and OBIEE) and self-serve reporting platforms such as Tableau, Qlikview, or Domo.
The Machine Intelligence Maturity Stage
As more data is generated, BI starts to open doors for MI. Although BI is always looking into the past and is highly comparative, machine intelligence looks at patterns from the past to predict future trends, which improves the decision process. Another aspect that machine intelligence brings to the table is its ability to look at complex relationships across multiple business parameters and determine the possible root cause(s) of past issues without human bias. The potential impact is higher and more strategic. However, a critical aspect for the success of machine intelligence is the data needed to make accurate estimations.
Impact to business: High
Cost to business: High
Consumers: Upper-middle to upper management
Analysts ask questions to solicit predictions or ask about data in complex relationships, such as:
- What internal and external factors can be attributed to a fall in sales last year?
- Based on historical failures of industrial assets, can we predict the same in the future and thus save production loss?
- Out of a myriad of sensor parameters, can we correctly determine the root cause of failure?
Machine intelligence systems are heavy on resources -- people and processing power. They need skilled data scientists to work with large servers that can crunch millions of records and surface little diamonds in return. This is supported by big data setups that can store large data volumes and machine learning methodologies that can run at scale. However, big data setups are not compulsory if the existing infrastructure can support the data volumes being generated. This strengthens the case for movement to the cloud so the infrastructure complexity and ad hoc requirements for processing power can be reduced.
The Artificial Intelligence Maturity Stage
The most strategic and data-hungry intelligence is artificial intelligence. Although machine intelligence is focused on providing better inputs for humans for decision making, AI makes decisions by itself, hence its name. The need for AI stems from the fact that there are certain processes that can be automated to a point that they require minimal or no human intervention. This improves efficiency, reduces personnel cost, and increases productivity. The impact is transformational. However, it requires time and a high degree of confidence to be put into production.
Impact to business: High
Cost to business: High
Consumers: Unit heads
Example processes for AI in manufacturing include:
- Quality control (such as inspection of faulty parts in factories through image recognition)
- Robotic arms to build parts using CAD diagrams automatically
- Predictive maintenance based on machine conditions
AI requires extremely sophisticated deep learning methodologies to learn from past behavior and create a decision model. The processing power needed to run deep learning algorithms is high and may even require GPU setups to be performed at scale. It's an iterative process so the time to production is long because the complete process cannot be left alone unless it has been thoroughly tested. Moreover, due to the development and training costs, implementing AI applications at scale requires a visionary mindset from the management team and deep pockets for R&D.
A Final Word
Data is only as good as the way it is used. A successful data strategy starts with the business problem and then rolls down to the technology that is needed to solve the problem. It's easy to get carried away with all the noise around cool technologies, but it takes time and maturity to get the most out of it.